At the world's top computer vision conference, Lenovo won 6 championships!
Computer vision is one of the significant technological fields in artificial intelligence. Each year, numerous academic and industry conferences on computer vision are held both domestically and internationally. Among them, the IEEE Conference on Computer Vision and Pattern Recognition (CVPR), the International Conference on Computer Vision (ICCV), and the European Conference on Computer Vision (ECCV) are the three most renowned top-tier conferences.
While facilitating global scholarly exchange and discussion, these top conferences also host a series of challenge competitions, attracting many top teams from around the world to actively participate. They compete in various subfields of computer vision, showcasing their skills and striving for excellence.
The CVPR conference held in late June is no exception. In the various computer vision challenge competitions organized during this conference, the Lenovo Research Institute team won a total of six championships, including:
- The 4D Vision Challenge (Ego4D and EgoExo4D Challenge) Social Interaction (Looking At Me) track champion;
- The 4D Vision Challenge (Ego4D and EgoExo4D Challenge) Hand Pose track champion;
- The Autonomous Driving ARGOVERSE Challenge 3D Object Detection track champion;
- The Autonomous Driving ARGOVERSE Challenge 3D Multi-Object Tracking track champion;
Advertisement
- The Autonomous Grand Challenge (AGC) Embodied Multimodal 3D Visual Grounding track champion, and also received the Most Innovative Award;
- The AI City Challenge Multi-Camera Multi-People Tracking track champion.
In the competition, the PC Innovation and Ecosystem Laboratory team of the Research Institute won the first four championships, while the Artificial Intelligence Laboratory, in collaboration with Tsinghua University and Shanghai Jiao Tong University, secured the championship in the embodied multimodal 3D visual localization of the Autonomous Systems Challenge, the Most Innovative Award, and the championship in the multi-camera multi-pedestrian tracking track of the AI CITY Challenge.
The 4D Vision Challenge integrating first-person perspective and external perspective (Ego4D and EgoExo4D Challenge)
The Ego4D dataset is a large-scale, egocentric video dataset and benchmark suite. It provides 3,670 hours of everyday activity videos, covering hundreds of scenarios (home, outdoor, workplace, leisure, etc.), filmed by 931 unique camera wearers from 74 locations worldwide and 9 different countries.
The Ego-Exo4D dataset, on the other hand, is a diverse, large-scale multimodal multi-perspective video dataset and benchmark suite. Ego-Exo4D captures both egocentric and exocentric, widely recognized human activity videos (such as sports, music, dance, bicycle repair, etc.).
Based on these two datasets, CVPR2024 proposed a series of new benchmark challenges centered around understanding first-person visual experiences. The PC Innovation and Ecosystem Laboratory team of the Research Institute won two championships in the social interaction (Looking At Me) track and the hand pose estimation (Hand Pose) track.
Social Interaction (Looking At Me) Challenge
In the social interaction (Looking At Me) track, the team won the championship with a score of 80.91 mAP (mean Average Precision).
Social interaction is key to understanding human behavior. By obtaining egocentric video data, we can gain a unique perspective that captures verbal communication and non-verbal cues of each participant. This technology provides a valuable source of information for studying social interactions, helping to deeply understand human social behavior. In the future, this technology is expected to promote the development of virtual assistants and social robots, enabling them to better integrate into human social environments and provide smarter, more considerate interaction experiences. By analyzing the subtle signals of social interactions, we can cultivate artificial intelligence systems that are more empathetic and socially intelligent, allowing them to communicate and interact more naturally with humans.
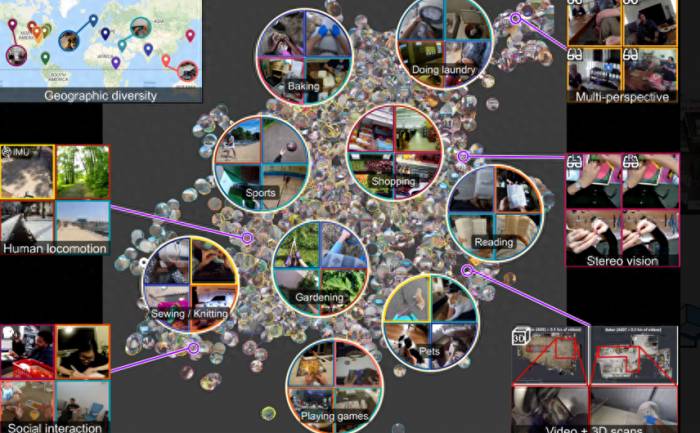
For example, this technology can be used to detect the emotional state of family members and provide suggestions or play music to alleviate emotions, with situational understanding and response capabilities. Furthermore, when a smoke alarm is detected in the kitchen, it can not only immediately notify family members but also automatically contact emergency services and guide children in the home to evacuate safely.
In this challenge, participants were given a video that included faces of social partners that had been localized and identified, and they were tasked with classifying each visible face to determine whether they were looking at the camera wearer. The task is highly challenging due to the distance between people and the camera in the scene, as well as human movement, which causes blurring of the facial images.Facing this challenge, the team proposed a solution called InternLSTM, which consists of an InterVL image encoder and a Bi-LSTM network. The InterVL is responsible for extracting spatial features, while the Bi-LSTM extracts temporal features. To address the complexity of the task, we introduced a smoothing filter to eliminate noise or spikes in the output.
Hand Pose Estimation Challenge
In another track of the CVPR2024 First-Person Perspective and Egocentric 4D Vision Challenge—Hand Pose Estimation, the team achieved the first place in the challenge with a score of 25.51 MPJPE (Mean Per Joint Position Error) and 8.49 PA_MPJPE (Procrustes Aligned MPJPE).
In this challenge, the team was required to accurately capture and reconstruct the 3D pose of the hand from video images taken from an egocentric perspective, including precise estimation of 21 3D joints. This not only demands ultra-high precision from the algorithm but also a deep understanding of complex hand poses.
Due to the subtlety of hand movements and frequent occlusions, this task is extremely challenging. To handle this complex task, we proposed a Transformer-based 3D hand pose estimation network (HP ViT). HP ViT includes a ViT backbone network and a Transformer decoder, using MPJPE and RLE loss functions to estimate the 3D hand joint positions.
Our ViT-Huge model was trained 20 times using the MPJPE loss function, and then fine-tuned with the RLE loss function to further enhance performance. We found that fusing models trained with different hyperparameter settings can reduce overall error.
Next, we plan to extend this pose estimation method from single images to video sequences, integrating hand motion information to further improve model performance. Through these strategies, we hope to continue optimizing the model to provide a more accurate and powerful solution for pose estimation tasks.
3D hand pose recognition technology can empower various scenarios. For example, in a VR shooting game, players can simulate shooting actions by physically waving their hands, and the game executes corresponding shooting commands by recognizing hand poses. This technology can also be used to assist people with disabilities by controlling wheelchairs or other assistive devices through hand motion recognition, improving their quality of life. In the medical field, 3D hand pose analysis can also help doctors assess patients' rehabilitation progress and provide personalized rehabilitation training plans.
Autonomous Driving ARGOVERSE 3D Object Detection & 3D Multi-Object Tracking ChallengeArgoverse 2 is a collection of open-source autonomous driving data and high-definition (HD) maps from six cities in the United States. This version builds upon the initial release of Argoverse ("Argoverse 1"), which was one of the first datasets of its kind to include HD maps for machine learning and computer vision research.
In the Autonomous Driving ARGOVERSE 3D Object Detection and 3D Multi-Object Tracking competition, the team designed an end-to-end unified perception prediction scheme called Le_E2E_Forecaster, integrating various sensors, including lidar and 360° surround camera inputs, and enhancing features with historical information. They used a Deformable DETR decoder to simultaneously handle multiple subtasks such as detection, tracking, motion prediction, and occupancy network prediction.
Ultimately, on the 3D Object Detection track, they achieved a 43% score in the Corner Distance Similarity (CDS) metric, which is 16% higher than the second place. On the 3D Multi-Object Tracking track, they scored 64.6 in the Higher-Order Tracking Accuracy (HOTA) metric, which is 5% higher than the second place.
3D object detection and tracking technologies are widely used in autonomous vehicles, capable of real-time recognition and tracking of the positions and velocities of surrounding objects, such as pedestrians, other vehicles, and traffic signs. For example, in urban traffic environments, this technology can assist autonomous driving systems in making safe decisions, such as avoiding pedestrians or changing lanes. Additionally, 3D object detection is also used in drone navigation, where drones can automatically plan flight paths by recognizing terrain and obstacles, enabling precise cargo delivery or terrain mapping.
Autonomous Systems Challenge: Embodied Multimodal 3D Visual Localization
In the "Multi-View 3D Visual Grounding" track of the CVPR2024 Autonomous Systems Challenge, the AI lab of the research institute, in collaboration with Tsinghua University, outperformed international and domestic universities such as Harvard University, École Polytechnique Fédérale de Lausanne, The Chinese University of Hong Kong, and University of Science and Technology of China, as well as companies like Microsoft and Xiaomi, winning both the championship and the most innovative award.
Compared to general AI, Embodied AI places greater emphasis on integrating artificial intelligence into physical entities such as robots, thereby enabling robots to perceive and understand their environment and interact dynamically with it. Embodied multimodal 3D visual localization is an important field related to Embodied AI technologies.
This challenge focused on indoor scenarios. Compared to common 3D perception tasks, indoor 3D perception systems face more challenges, such as multimodal inputs (including images, 3D point clouds, and language instructions), a more diverse range of object types, the need to pay attention to different object categories and orientations, even their relative positions, and more complex spatial scenes.
The main challenges of this task include: multimodal input information (3D point clouds, images, language), especially the addition of the language modality, which greatly increases the difficulty of the task; and the detection of small-sized indoor objects in the 3D point cloud modality. In response to these two difficulties, the team proposed the following solutions:Language Modality Enhancement: The image above is a data sample. The task requirement is "find the chair next to the table," but there are actually many chairs in the image, with only one being "next to the table," which can greatly interfere with the model's prediction. To address this issue, the team used a Large Language Model (LLM) to enhance the original text data, thereby constructing richer semantic information.
Through the steps illustrated in the image above, a simple description like "the chair next to the table" can be transformed into "the chair next to the table, closest to the television and farthest from the window," allowing the model to more easily locate the target object.
Multimodal Fusion: Another challenge of this task is that objects in indoor scenes are too small, making it difficult for point cloud data to capture small targets, such as the mouse in the scene below, from which the LiDAR can only obtain minimal signals; however, for the camera, obtaining the position of the mouse is much easier.
Traditional multimodal fusion typically involves first merging information from images and point clouds, and then merging it with text information. The drawback of this fusion method is that the model does not know which parts of the 3D space to focus on. Taking the mouse as an example, directly fusing image and point cloud information may not help in detecting the mouse and could even weaken the signal of the mouse in the 2D image.
In response to this situation, we have designed a new multimodal attention mechanism with the overall framework as follows:
We first fuse multi-perspective image information and text information through a module called Bi-TVI, which aims to guide the network to focus on the "truly necessary parts" through attention mechanisms. After the attention interaction, the image features carrying attention information are then fused with the 3D point cloud information, thereby achieving efficient detection of small-sized indoor objects.
AI City Challenge Multi-Camera Multi-People Tracking
The AI City Challenge is one of the most renowned competitions in the field of intelligent transportation internationally. This year, in the multi-camera multi-pedestrian tracking track of the event, the joint team of the research institute's artificial intelligence laboratory and Shanghai Jiao Tong University won the championship.
The main task of the multi-camera multi-pedestrian tracking track is to detect and track each person in occlusion scenes across cameras and assign the same ID to the same object on different cameras. This year, the track significantly increased the difficulty of the data: the number of cameras increased from 129 to around 1300, and the number of pedestrians increased from 156 to around 3400. At the same time, to encourage participants to use online algorithms, online tracking will receive an additional 10% bonus score.Based on the project scenario, the team has designed an online tracking system based on appearance consistency and spatial consistency. The system integrates spatial information within and between cameras, as well as adaptive appearance information of the targets. When matching the detection results from multiple views with the tracking targets, it simultaneously considers 2D spatial information, 3D extreme distances, homography distances, and adaptive Re-ID similarities. The first three are aimed at meeting the geometric constraints within a single view and between different views, while the latter helps to correct ID switch issues during and after severe occlusions. To avoid multiple trajectories caused by significant Re-ID differences between the same ID individual at different viewpoints, the team has specifically designed a Re-ID feature repository to store Re-ID features corresponding to different poses and angles, giving the system a strong online ID re-identification capability, which is extremely important in scenarios with dense crowds and severe occlusions.
In recent years, the Lenovo Research Institute has been committed to the development of multimodal visual perception and large language models/multimodal large models. Winning six championships this time fully demonstrates the team's technical capabilities in these areas. In the past few years, in the competitions organized by top conferences in computer vision, including CVPR and ECCV, the Lenovo Research Institute team has repeatedly won championships in multiple tracks.